The Duplicator
Imagine a magical cardboard box capable of duplicating people- their memories, skills, and personalities. Calvin didn’t have to imagine such a box. The comic-strip comic invented one in an attempt to get out of cleaning his room in the beloved children’s series,Calvin & Hobbes.
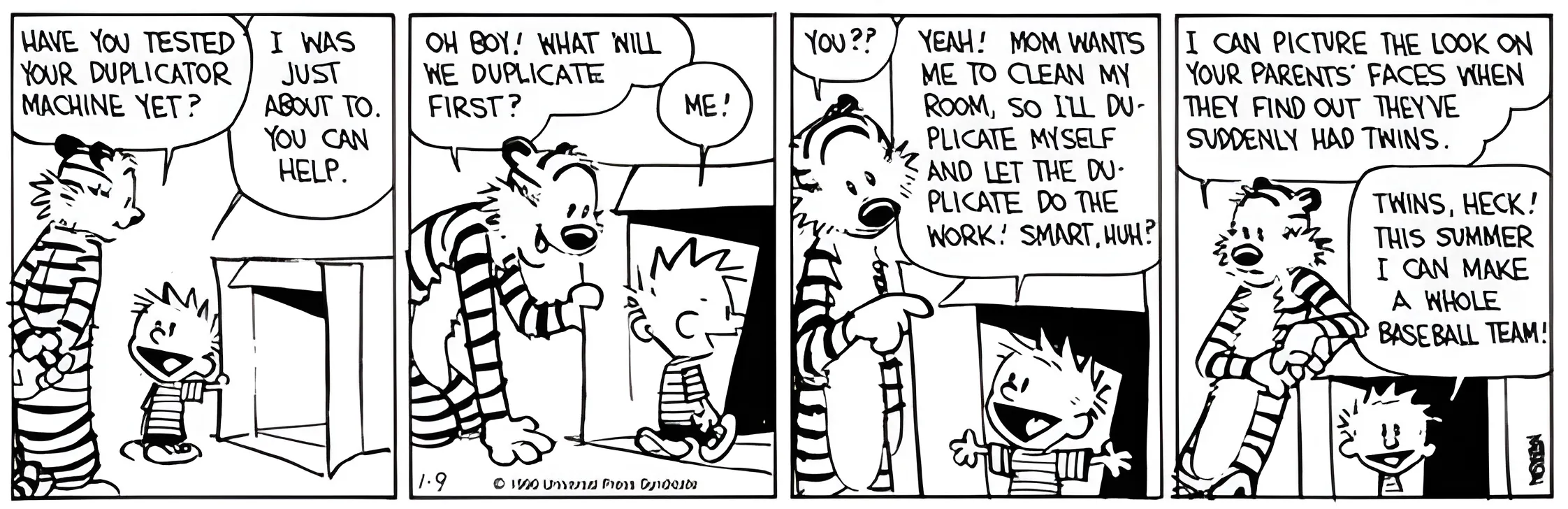
Calvin & Hobbes’ “Duplicator”
Imagine if Calvin’s duplicator came into existence tomorrow. How would we use it? Would we use it to try to get out of cleaning our rooms? The most compelling use might be to duplicate our brightest minds from leading academic institutions. What would happen if we could replicate our most talented scientists indefinitely? How would the world change with unlimited access to their combined intellect and labor?
Open Philanthropy’s Holden Karnofsky (who I borrowed this thought experiment from) argues that the ability to mass-manufacture our brightest and most creative minds would catalyze explosive technical and economic growth. Karnofsky discusses the duplicator as a metaphor for a technology capable of alleviating the bottleneck between population growth and technical/economic progress.
I think the Duplicator would be a more powerful technology than warp drives, tricorders, laser guns¹² or even teleporters. Minds are the source of innovation that can lead to all of those other things. So being cheaply able to duplicate them would be an extraordinary situation.” – Karnofsky, in “The Duplicator”
The removal of this bottleneck tying technical progress to population growth will leave science, and the world, looking fundamentally different. The impact of the duplicator is intuitive. The runaway creation of scientific talent will lead to runaway technical development, but the path to a real-life duplicator is much less intuitive. How do we get there, and why should we think about getting there?
AI Scientists: Real-Life (or soon to be) Duplicators
We cannot physically clone the minds of existing human scientists, but large language models are becoming increasingly capable of completing many of the cognitive tasks that underlie the scientific method, including hypothesis generation, experiment execution & troubleshooting, and knowledge distillation. These developments have occurred at breakneck speed. However, these models are limited in silica, especially for discovery in the physical sciences. Unlike human scientists, they cannot interact with the world and experiment to test and refine their hypotheses. Biology will not be solved in silica soon; rather, it will require experimentation and the collection of massive amounts of reproducible data to enable more robust simulations. Combining language models with lab robotics provides them with the agency to physically interact with the world to progress the scientific method similar to human scientists. The vision of the duplicator and unlimited scientfific talent will be achieved through combining these models with physical agency, enabling them to interact and experiment in the physical world. Recent pioneering work in academia (ChemCrow⁴, Organa⁵, and Coscientist⁶) have demonstrated a proof-of-concept for this idea. The first major impact of this work, and necessary prerequisite towards AI scientists, will be the development of assistants that abstract the physical execution of experiments, to allow human scientists to focus on hypothesis generation and data analysis. However, the first step to achieving this ambitious vision is bridging the communication gap between these AI scientists and humans.Step One: Bridging The Communication Gap
Many of us remember the cliche first programming lesson. You are asked to detail how to make a peanut butter and jelly sandwich. Maybe you say “put some peanut butter and jelly on some bread and put the slices together”. The instructor, invariably, will poke holes in your instructions no matter how granular they are. How many grams of peanut butter? How spread out should it be? What sides of the bread should the butter and jelly go on? The lesson here is that computers run based on an explicit set of instructions, whereas we operate based on implicit instructions. “Go make a peanut butter and jelly sandwich” is a high-level, abstract command that we take and turn with our minds into actions.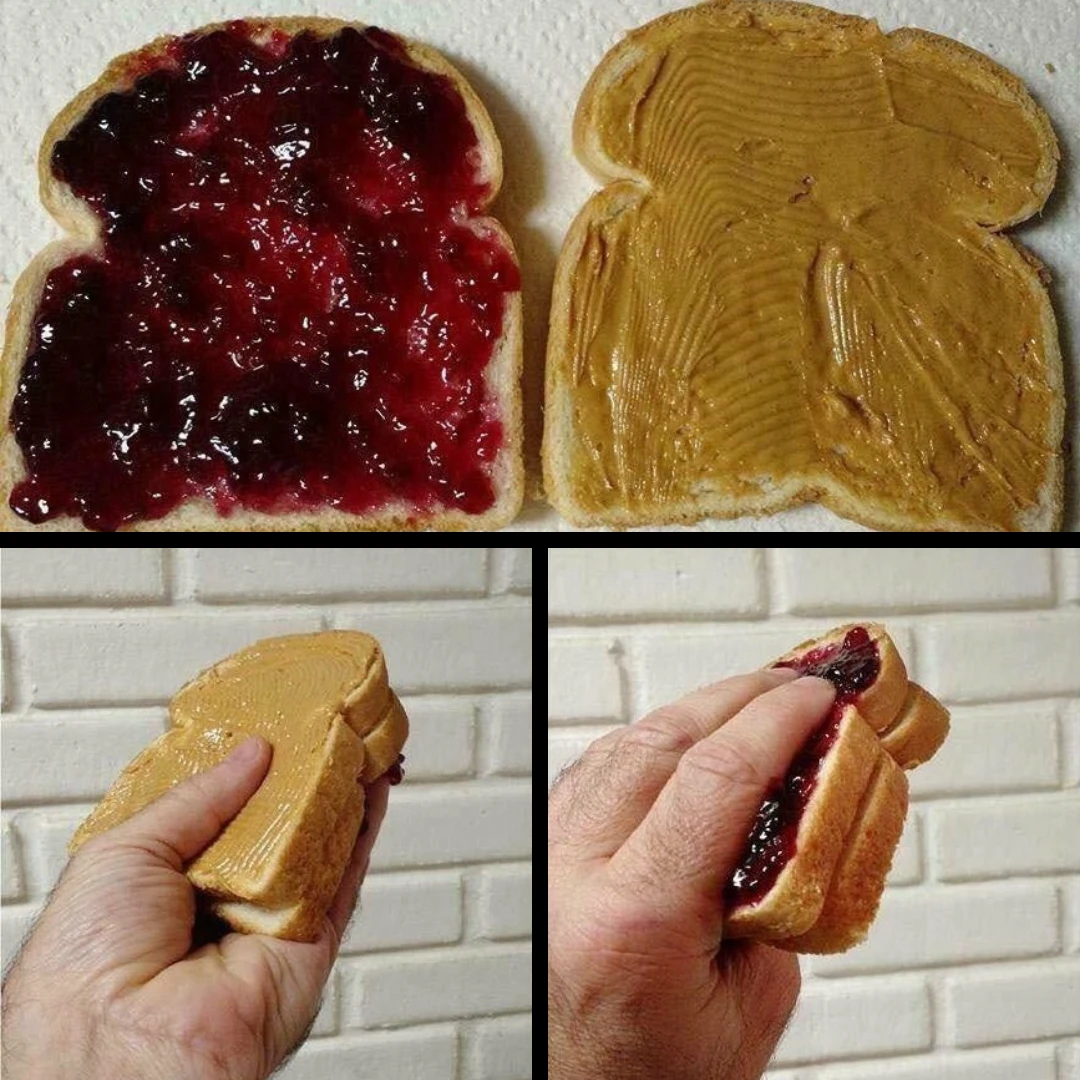
Computers (traditionally) lack the functionality to turn these same high-level instructions into detailed, non-ambiguous commands. We program computers with detailed, non-ambiguous commands via programming. Similarly, we program lab robots with very detailed, non-ambigous commands. In addition to requiring programming knowledge, this work also requires a niche set of scientific knowledge, ranging from the properties of different liquids and how they impact pipetting to the optimal labware to use for a workflow (amongst many other things). Unfortunately, scientists lack much of the programming knowledge required to translate their own intent into a computer program, and programmers lack the scientific knowledge to create such programs.
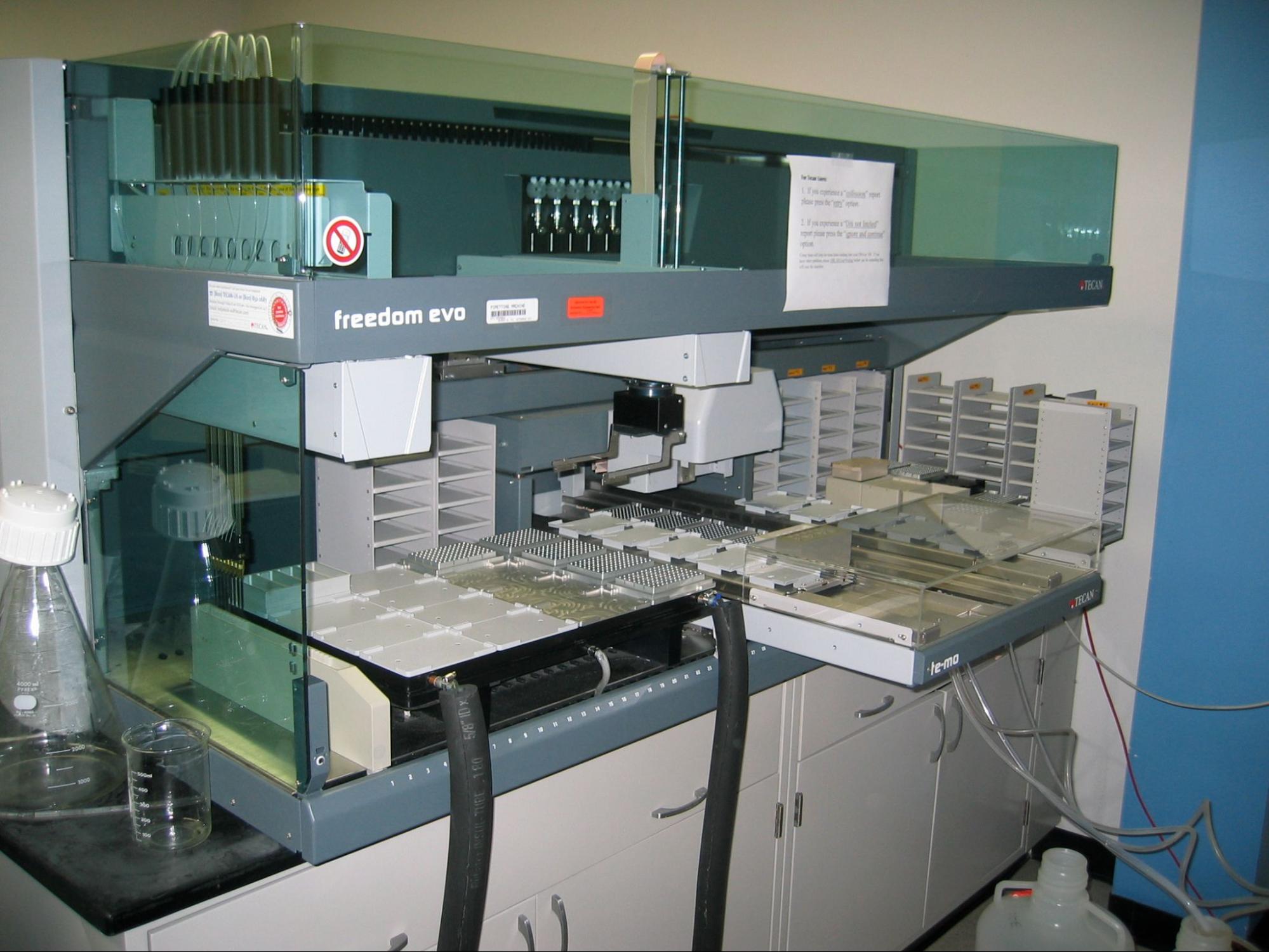
A pipetting robot (known as a liquid handler), the foundational lab robot
A good way to think about how AI scientists can augment scientific labor is to think about cooking. No one who goes to culinary school and becomes an expert in molecular gastronomy wants to be a line cook. In the life sciences, too many of our chefs today spend time as line cooks. In this analogy, the tools we are developing resemble line cooks, performing the repetitive physical labor to allow chefs to focus on improving current dishes or cooking up new ones. But what if chefs couldn’t actually communicate with their line cooks? What if these line cooks not only couldn’t speak with chefs, but had no concept of cooking and had to be painstakingly taught the movements and steps to making each dish by a specialist who could speak both their language and the chef’s? They would probably only be good for making a billion pizza rolls in a factory, instead of being helpful to chefs in restaurants. Unfortunately, this analogy represents the lab automation tools of today, drastically limiting their applications and usefulness.
This communication problem belongs within the realm of a relatively small, old industry known as lab automation. This industry relies on a specialist engineer known as a lab automation engineer to convert the intent of scientists into robotic code. This profession draws on an incredibly diverse range of knowledge, ranging from mechanical engineering to bioengineering and programming. The high-activation energy process of performing the translation of intent into robotic code is only justified for high-throughput, low-variety tasks that resemble manufacturing. It cannot be justified for the high-variety experimentation many scientists rely on at the bench. As a result, today’s lab automation tools are highly limited in use case and usefulness. Solving the communication gap to lower the activation energy to use these tools is the first step to building AI scientists that empower scientists to innovate faster and more reproducibly.
Why Work Towards This?
Like many in the life sciences, I have been motivated to pursue this path by a desire to create things that would have helped people I love. However, my brief time at the bench taught me that while the promise of innovation in the life sciences is great, the path to that innovation is not. Science today is brainpower inefficient: we spend far too much time at the bench and in front of excel sheets, instead of talking with colleagues or reading literature to come up with new ideas. Researchers in life science often lack the reliable abstractions that our colleagues in engineering rely on to focus on higher level ideation. What would aerospace or civil engineering look like if no simulators existed and everything was integrated by hand (and many people made mistakes or had bad handwriting that made the work hard to reproduce)? Mass-producing the scientific labor responsible for the execution of experiments will allow us to perform more experimentation, more reproducibly. More than ever before, scientists will be empowered to do real science, not mind-numblingly repetitive tasks. This is our goal in the short to medium-term: To create assistants capable of interacting with human scientists as seamlessly as they interact with each other, to consistently perform the experimentation and repetitive work that we loathe. Human labor will be refocused on data analysis and hypothesis generation- real science. The life sciences will shift closer to being a discipline of engineering, not an artform that evokes superstition and varies with the hand of each artist. The longer term goal in pursuing the development of an AI scientist seeks to fulfill Karnofsky’s vision of a replicator that catalyzes hyperbolic growth. This cannot be done alone, if we work tirelessly and do everything right, we may be lucky enough to play a part in advancing humanity towards this grand goal. The impact we hope for our work to have may sound deranged for a team of 20-something year olds who just raised a pre-seed round, but our reasoning is as follows:- In the short-to-medium term, advances in artificial intelligence applied to lab automation will result in tools that are massively more valuable to scientists. Specifically, advances in natural language processing will bridge the communication gap between scientists and tools that traditionally required a specialist engineer, making these tools much more flexible and enabling their use for high-variety workflows.
- In the long term, AI scientists will be capable of more complex and abstract tasks, such as hypothesis generation and knowledge distillation. Our focus is less on this topic, and more on building what we consider to be the prerequisite steps for these concepts to be useful for a physical lab automation platform.
- The marriage of a platform that can communicate with scientists and physically execute experiments with models capable of hypothesis generation and knowledge distillation will result in an AI scientist with capabilities that represent a PhD researcher. This is the duplicator.
- The emergence of an AI scientist and automation of the scientific method is functionally the same as the emergence of a duplicator. The mass-production of these systems will catalyze an unprecedented rate of scientific progress.
- The first step in reaching the duplicator is to bridge the communication gap. Recent advances in NLP/language models make this possible for the first time ever. This is one of our primary focuses.
The Journey of a Thousand Miles
Tetsuwan is excited to announce an oversubscribed pre-seed round, led by 2048 Ventures. We are also thankful for participation from Carbon Silicon, Everywhere, Referent Ventures, 100 Plus Capital, Neman Ventures, Transpose Platform, and our friends at Entrepreneur First. This capital has enabled rapid growth, as we have developed into a seven-person team coming from across Caltech, MIT, ETH-Zürich, UC Davis, and UCSF. This funding will not only benefit our team, but also our first few deployments as we seek to rapidly build, break, and iterate. I am writing this post from a lab bench in San Diego, where we are currently testing our first system at a rare disease RNA therapeutics company. It is a baby step towards our grand vision, but nonetheless, will work to serve scientists and empower them to innovate. A mentor of mine reminds me, “A life is not important except in the impact it has on other lives”, a quote from the late Jackie Robinson. Our work in developing these tools and attempting to catalyze hyperbolic innovation is not important except in the impact it has on other lives. Many things may change post-hyperbolic growth, but our commitment to do good cannot be one of them.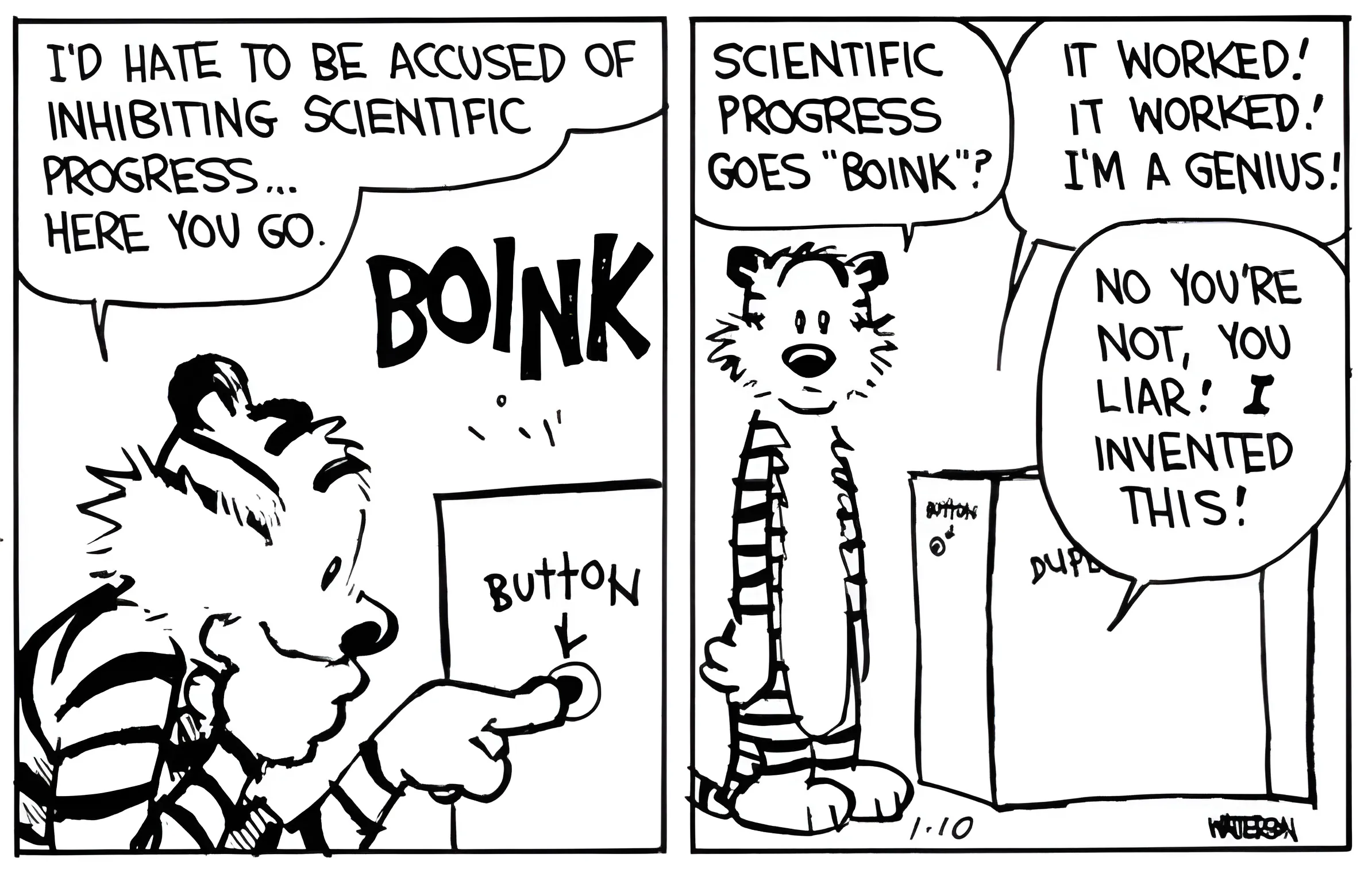
¹ Thank you to Zero Shot’s Laszlo Kopits & Robert Miles from Rational Animations for helping me find this thought experiment!
² Another path to augmenting the relationship between population growth and technical progress is to automate scientific discovery itself, instead of mass-producing minds to advance discovery. Karnofsky refers to this technology as Process for Automating Scientific and Technological Advancement. I discuss soon that I believe that the in silica replication of minds combined with physical agency is the most obvious path towards a PASTA.
³ Noted in Sakana’s AI scientist discussion section.
⁴ ChemCrow
⁵ Organa
https://www.cold-takes.com/transformative-ai-timelines-part-1-of-4-what-kind-of-ai/